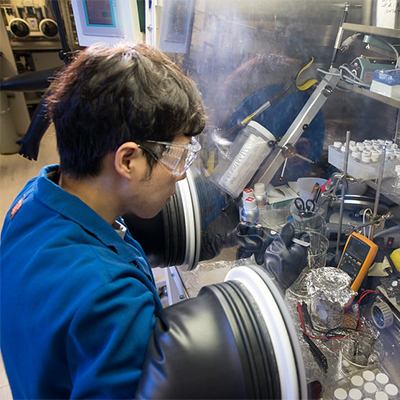
An Argon-filled “glove box” in the IBM Research Battery Lab, which is used to prepare air-sensitive battery materials such as lithium metal anode and electrolyte formulations, both of which were used in this new battery design. Courtesy: IBM Research
By Young-Hye Na, Manager of Advanced Battery Research Program, IBM Research-Almaden, US
Our world has no shortage of problems to solve. We now stand at a critical juncture for global action to address our most pressing challenges; from the COVID pandemic to climate change and so much more.
IBM has long recognized the urgency to find more sustainable solutions to tackle these problems (The Urgency of Science). For the first time in history we have the right tools at our disposable to do so. AI (artificial intelligence)—combined with advanced computing and access to enormous volumes of data via a secure and open hybrid cloud—can significantly accelerate the process of scientific discovery and the creation of more sustainable materials for use across a broad range of industries, including energy and batteries.
Better batteries for cleaner energy
The energy sector is responsible for more than 70 percent of man-made greenhouse gas emissions worldwide. Therefore, a prompt transition toward cleaner energy to reduce our dependence on fossil fuels is a critical priority to address climate change. Reliable use of renewable energy requires the ability to store intermittent energy such as solar and wind. Right now, the world can store only about 3 percent of the electricity produced globally. To limit global warming to below 2 degrees by 2050, we have to triple the amount of energy we can store. The growth of the electric vehicle (EV) market is also dependent on the availability of cheaper, safer, and more powerful batteries. In short, the world needs better batteries.
Lithium-ion battery (LIB) technology—still king when it comes to energy storage—is considered one of the most efficient and lightest battery solutions. However, there are still many challenges surrounding EV batteries and battery energy storage systems, from the gradual saturation in achievable battery performance to their accessibility and sustainability. Heavy metals that are needed to make these batteries—such as cobalt and nickel—are difficult to mine, limited in quantity, and harmful to the environment if they are not disposed of properly. What’s more, cobalt is mainly found in the Democratic Republic of Congo, where human rights groups have long raised concerns for careless and exploitative human labor practices to extract these metals.
Still, lithium-ion batteries are gradually getting more efficient, with prototypes sporting relatively low cobalt content and/or solid state electrolytes aimed at improving battery safety and energy density. But, at IBM Research, we are also looking at next-generation batteries, betting on emerging computational technologies including artificial intelligence (AI) to help us find the necessary materials.
AI for accelerated discovery of battery materials
AI, much like a scientific instrument or a piece of technical equipment, is a tool that has the potential to accelerate progress in a number of areas important to sustainable battery design, such as faster charging, lower cost, and higher sustainability.
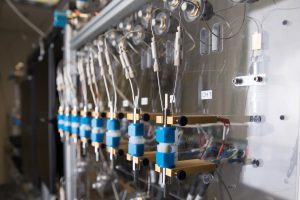
A Differential Electrochemical Mass Spectroscopy (DEMS) System in the IBM Research Battery Lab, which measures the amount of gas that has evolved from a battery cell during charging and discharging cycles. Courtesy: IBM Research
Through our own research, our team at IBM Research has developed a high power, fast-charging battery that is free of heavy metals. AI has the capability to help us advance the performance of this battery chemistry, and can help to get the best performing version of this battery to consumers as soon as possible. For example, we are using AI techniques to discover safer and higher-performing electrolyte candidates that can increase the safety of our battery. These approaches use semantic research combined with Expert-in-the-Loop AI models.
While the role of semantic search is to evaluate the potential usability of solvents reported in literature, AI complements this by capturing institutional knowledge and domain expertise of subject matter experts and directing the semantic search in a manner that increases relevance and actionability of search results. Solvent candidates identified by AI move to the experimental stage, and advanced data acquisition strategies (such as active learning and Bayesian optimization) help to reduce the required volume of experiments needed to find safer and higher performing systems.
Additionally, IBM global research teams have designed extremely effective and useful AI models to accelerate materials discovery across the entire life cycle of the discovery process—from materials search to the final synthesis. This is applicable to any technology sector, and useful tools include:
- Deep search algorithms to quickly pull knowledge from tremendous volumes of existing scientific literature;
- Algorithms to efficiently decide which and what kind of simulations to run to better understand materials and their functions;
- Generative models to rapidly suggest new materials;
- AI-driven autonomous lab processes which can assist human researchers with automated and custom synthesis of materials.
All of this ladders into one ultimate objective: supercharging the discovery of materials to enable a more sustainable future. Materials design and discovery with AI has a critical place in our economy, and speeding up scientific processes to discover these new materials (as well as rethink how we consume and reuse current ones) can help us solve urgent and pressing global issues around energy and batteries, as well as other industries such as health, farming, and transportation.
Please visit IBM’s 5in5 Accelerated Material Discovery for more details.